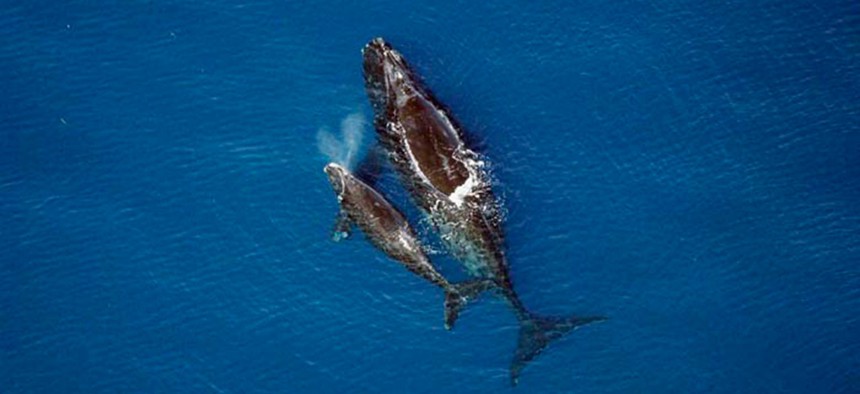
NOAA
Why NOAA is Working on a Facebook for Endangered Whales
Marine biologists have crowdsourced a facial-recognition algorithm to help them identify the animals on the spot.
There are only around 500 North Atlantic right whales left in the world, making them one of the most endangered of all whale species. This month, nearly that many data scientists raced to complete a project that might help researchers keep this small population from disappearing altogether. Their goal: Develop an algorithm that could identify any living North Atlantic right whale from a photograph of its face.
The contest was the brainchild of Christin Khan, a biologist at the National Oceanic and Atmospheric Administration’s Northeast Fisheries Science Center, who was looking for a way to solve a problem she and other whale researchers come across every day in their work. Khan is part of a team that flies aerial surveys over the waters off the U.S. East Coast to look for North Atlantic right whales. (Two other species of right whale live in the Southern Hemisphere and the North Pacific.) To keep tabs on their target population, researchers track all of the whales individually, using each animal’s distinctive facial markings to identify the ones they see swimming below. (Some even have names: Whale 1611 is Clover, whale 1006 is Quasimodo, whale 1250 is Herb.) But the process can be difficult and tedious.
When the aerial-survey group spots a whale, they open the back window of their Twin Otter plane and snap a photo. Some whales are so distinctive that the team knows them by sight—but more often, the researchers don’t know who they’ve spotted until they get back to the office and consult the North Atlantic Right Whale Catalog. Maintained by the New England Aquarium, this online database has photos and detailed drawings of almost 700 right whales, alive and dead. Researchers study the photos they’ve taken at sea, input certain features of the whale, and then comb through the possibilities until they find a match.
Khan makes about 60 survey flights a year, covering roughly 25,000 square miles of ocean from Maine to south of Long Island—the main U.S. feeding grounds of North Atlantic right whales. A tricky identification from the catalog can take hours, she says. So after a flight that finds, say, a hundred whales, identifying all of them can be an exercise in frustration, not to mention a time suck.
On one such tedious day in the office a few years ago, Khan took a break from poring over whale images to log into Facebook, where she was greeted with a notification asking her to confirm that she was in a photo. As Khan tells it, the moment was an epiphany: Facebook’s algorithms had identified her face. Why couldn’t she have something similar for whales?
Khan first contacted Facebook itself, asking if the company was interested in a little pro-bono work to support endangered whales. (It wasn’t). After trying some other avenues, she thought of Kaggle, an online platform that hosts data-science competitions. The site came recommended by a colleague, the Cornell University scientist Christopher Clark, who’d used a Kaggle contest to successfully solicit an algorithm for detecting right-whale calls in audio recordings.
Khan’s dream, she told the team at Kaggle, was an algorithm that could scan any photo of a right whale—whether taken from a plane overhead, or a vessel alongside the animal—and use it to identify the animal. But since these two processes represent very different challenges from a computer’s perspective, she ultimately scaled back the request, aiming for just an algorithm that could identify whales from overhead shots. Using several thousand labeled aerial photos of North Atlantic right whales, competitors had to write an algorithm that could identify who was who. The software company MathWorks offered to sponsor the competition, putting up $10,000 in prize money and free software for competitors—all Khan had to supply were the photos.
The contest kicked off at the end of August 2015. A total of 364 teams entered, made up of 470 players. Under the terms of the competition, they had until January 7, 2016, to refine their algorithms.
The first obstacle they faced was getting a computer to correctly zero in on each whale’s head. The pattern of whitish markings, or callosities, on a whale’s head is key to identifying it—but in an aerial photo, white bits of ocean spray can look remarkably similar to these spots. (One of the competitors, Artem Khurshudov, described his struggles with this part of the process in a blog post titled “Wrong Whales.”)
Throughout the contest, Khan monitored the Kaggle forums, where competitors were sharing ideas and frustrations. After one poster asked, “Do those white thinger-doodles change over time?” Khan posted some tips and basic terminology for whale identification: the white thinger-doodles are callosities; the nose end of the whale is its bonnet.
“Is the whale in w_8026.jpg pooping?” asked another competitor.
“Yes!” Khan responded. “We marine biologists refer to it as defecation.”
The competition’s winning entry, announced in early January, came from a team at the Warsaw office of the data-science company deepsense.io. Their algorithm could identify whales with 87-percent accuracy.
One of the team’s core members, the data scientist Jan Kanty Milczek, says the challenge was more similar to human facial recognition than he’d expected. After cropping a photo around a whale’s head, the next step was to get the computer to align it a certain way, with the whale’s blowhole on one side and its bonnet on the other, a process he likens to “making the passport photo of each whale.”
The team used a neural network, a kind of computer program that learns by example. The scientists trained the neural network to search for patterns among the photos, first on the scale of a few pixels, then with increasingly larger swaths of an image. “I think of it as more like giving hints to the actual algorithm than doing things for it,” Milczek says.
Khan says her next step will be to talk with other members of the right-whale research community and decide whether they should move ahead with creating software that uses the winning team’s algorithm, or solicit a second algorithm for identifying whales from vessel photos and then package the two together as a single piece of software.
Either way, Khan says, the deepsense.io algorithm could help researchers in several ways: Identifying a whale immediately would be useful to scientists doing biopsies of whales to study their genetics, for example. If they pull up alongside an animal and can identify it right away as one they’ve already tested, they won’t need to bother it a second time.
Then there are entangled whales, who’ve gotten caught up in bits of fishing gear and are dragging it from their bodies while they swim. When researchers spot an entangled whale, they contact a network of on-call disentanglement expertsalong the coast. Responders will jump in a boat, race to the scene, and decide whether to try to help the animal (some entanglements are life-threatening; at other times it’s better to leave the animal alone and keep monitoring it). If these responders can immediately identify an animal, Khan says, they’ll be able to pull up other photographs to get a better idea of how much gear it’s dragging, or how exactly that gear is attached.
But perhaps the biggest benefit of facial-recognition software for right whales, Khan says, is that it would free up researchers’ time to do actual research. Rather than spending long hours in the office clicking through the right-whale catalog, these chronically underfunded teams could use that time to collect data out in the field or work on papers for publication. Someday, similar software could even help the researchers who study other marine mammals—bottlenose dolphins are identified by their dorsal fins, for example, and humpback whales by their distinctive tail patterns.
In short, the software would buy researchers more time—and help the endangered whales they study have more time on the planet, too.