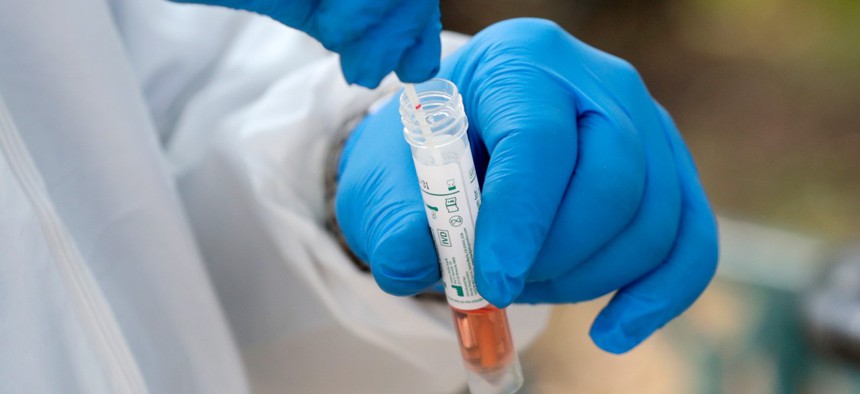
A medical worker places a swab in a vial while testing the homeless for COVID-19 through the Miami-Dade County Homeless Trust in Miami in April 2020. Lynne Sladky/AP file photo
Machine Learning System Predicts Severe COVID-19
An advanced machine learning system can accurately predict the risk of a patient's COVID-19 advancing to severe disease or death, say researchers.
An advanced machine-learning system can accurately predict if a patient’s bout with COVID-19 will become severe or fatal and relay its findings to clinicians.
Clinicians often learn how to recognize patterns in COVID-19 cases after they treat many patients with it. Machine-learning systems promise to enhance that ability, recognizing more complex patterns in large numbers of people with COVID-19 and using that insight to predict the course of an individual patient’s case.
However, physicians sworn to “do no harm” may be reluctant to base treatment and care strategies for their most seriously ill patients on difficult-to-use or hard-to-interpret machine-learning algorithms. The new system offers findings in an easily understandable form.
The prognostic tool, known as the Severe COVID-19 Adaptive Risk Predictor (SCARP), offers findings in an easily understandable form and can help define the one-day and seven-day risk of a patient hospitalized with COVID-19 developing a more severe form of the disease or dying from it.
As reported in a paper in the Annals of Internal Medicine, SCARP asks for a minimal amount of input to give an accurate prediction, making it fast, simple to use, and reliable for basing treatment and care decisions.
“SCARP was designed to provide clinicians with a predictive tool that is interactive and adaptive, enabling real-time clinical variables to be entered at a patient’s bedside,” says senior author Matthew Robinson, assistant professor of medicine at the Johns Hopkins University School of Medicine.
“By yielding a personalized clinical prediction of developing severe disease or death in the next day and week, and at any point in the first two weeks of hospitalization, SCARP will enable a medical team to make more informed decisions about how best to treat each patient with COVID-19.”
The brains of SCARP is a predictive algorithm called Random Forests for Survival, Longitudinal and Multivariate Data (RF-SLAM), described in a 2019 paper by its creators, Johns Hopkins Medicine researchers Shannon Wongvibulsin, Katherine Wu, and Scott Zeger.
Unlike past clinical prediction methods that base a patient’s risk score on their condition at the time they enter the hospital, RF-SLAM adapts to the latest available patient information and considers the changes in those measurements over time.
To make this dynamic analysis possible, RF-SLAM divides a patient’s hospital stay into six-hour windows. Data collected during those time spans are then evaluated by the algorithm’s “random forests” of approximately 1,000 “decision trees” that operate as an ensemble. This enables SCARP to give a more accurate prediction of an outcome than each individual decision tree could do on its own.
“The same way that individual stocks and bonds perform better as a portfolio—with the overall value staying strong as individual items balance each other’s rises and falls in price—the trees as a group create a flexible and adaptable forest that protect each other from individual errors,” Robinson says. “So, even if some trees predict incorrectly, many others will get it right and move the group in the correct direction.”
Most machine-learning systems used to make clinical prediction can only consider static data at a single point in time. “RF-SLAM enables us to be nimble and predict the future at any point,” Robinson says.
To demonstrate SCARP’s ability to predict severe COVID-19 cases or deaths from the disease, Robinson and his colleagues used a clinical registry with data about patients hospitalized with COVID-19 between March and December 2020, at five centers within the Johns Hopkins Health System.
The patient information available included demographics, other medical conditions, and behavioral risk factors, along more than 100 variables over time, such as vital signs, blood counts, metabolic profiles, respiratory rates, and the amount of supplemental oxygen needed.
Among 3,163 patients admitted with moderate COVID-19 during this time, 228 (7%) became severely ill or died within 24 hours; an additional 355 (11%) became severely ill or died within the first week. Data also were collected on the numbers who developed severe COVID-19 or died on any day within the 14 days following admission.
Overall, SCARP’s one-day risk predictions for progression to severe COVID-19 or death were 89% accurate, while the seven-day risk predictions for both outcomes were 83% accurate.
Robinson says he plans further SCARP trials to validate its performance on a large scale using national patient databases. Based on the results of the first study, Johns Hopkins Medicine has already incorporated a version of SCARP into the electronic medical record system at all five of its hospitals in the Maryland and Washington, DC, area.
“Our successful demonstration shows that SCARP has the potential to be an easy-to-use, highly accurate, and clinically meaningful risk calculator for patients hospitalized with COVID-19,” says Robinson.
“Having a solid grasp of a patient’s real-time risk of progressing to severe disease or death within the next 24 hours and next week could help health care providers make more informed choices and treatment decisions for their patients with COVID-19 as they get sicker.”
Source: Johns Hopkins University
This article was originally published in Futurity. Edits have been made to this republication. It has been republished under the Attribution 4.0 International license.