sponsor content What's this?
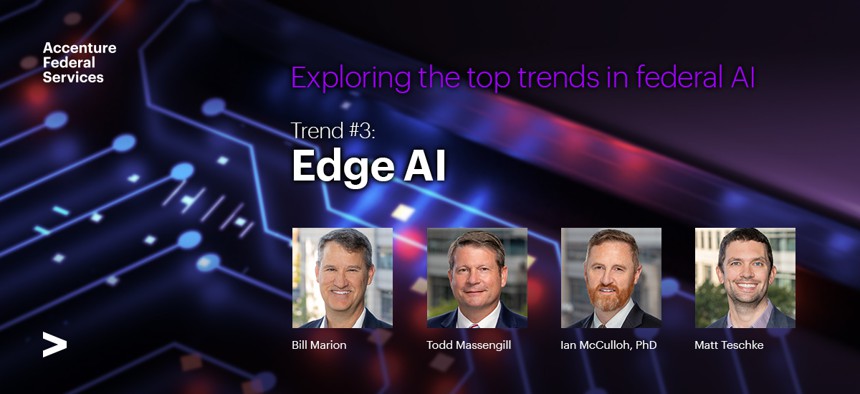
Edge AI offers advanced insight, when and where it’s needed
By embedding AI into edge computing devices, agencies can bring AI’s immense power out to the field and more reliably provide advanced insight no matter where the mission goes.
Presented by
Accenture Federal Services
Embedding AI into edge computing devices is one of the most powerful and exciting trends on the artificial intelligence (AI) landscape. So-called edge AI brings AI’s immense power to the field, where it can operate locally and independently to capture data, make computations, and produce actionable insight. This insight can then be used to guide and engage remote workers, or it can be implemented autonomously. When speed of decision truly matters, edge AI can be a gamechanger.
Edge AI isn’t a foreign concept. Just consider some everyday examples you’ve likely already encountered: Apple’s voice assistant Siri now analyzes audio directly on the device and can even operate some commands offline. Amazon’s Ring security cameras have the option to process and store video feeds locally. Our smart devices are increasingly using edge AI to optimize data processing for users depending on where the device is and what it’s being asked to do – letting them execute faster and more reliably.
Broadly speaking, edge AI excels in two primary use cases. First, it can help facilitate highly personalized experiences that users can interact with in real-time. For example, edge AI is foundational to delivering on the promise of augmented reality (AR), which can require low-latency, high-fidelity data processing. Second, edge AI is essential to the development of smart systems and environments, which are powered by vast amounts of data generated by proliferating Internet-of-Thing (IoT) devices. For example, consider the amount of data generated by emerging smart cities.
What’s more, the two use cases of personalized, real-time experiences and smart environments are starting to converge; individuals can use edge AI to interact with their current environments in dynamic, powerful ways.
Why we need edge AI
Edge AI is required because enterprises face a significant barrier as they work to unlock more value from AI across their operations: AI that requires routing through a centralized source can be limited by network connectivity and latency.
Consider this is the context of federal agencies’ increasingly distributed, complex missions. The flight of an F-35 fighter jet could be generating terabytes of data every hour while executing far-off missions. Sensors for crop monitoring may need to collect and process extensive data on remote farms. In these environments, reliable network bandwidth cannot be guaranteed and the cost for migrating all that data from devices at the edge to a centralized cloud may not be viable as the total amount of data continues to grow.
Edge AI, and edge computing more broadly, is being fueled by advancements in both network speeds, such as 5G, and available computing power. With edge AI, agencies can strategically deliver faster insight where it’s needed most, only passing data on for centralized computing if truly necessary.
This means edge AI can enable powerful intelligent systems that can sense, comprehend, and act independently. These can be physical manifestations, such as self-driving cars and autonomous robots. But edge AI can also be integrated into remote digital operations, such as for high-volume, high-fidelity data processing in distributed locations. Over time, these capabilities will become increasingly necessary. IDC predicts that by 2025, there will be 39.3 billion IoT devices, which will generate 74.4 ZB of data.
It’s good then that edge AI is already on many federal agencies’ radars:
- Accenture research found that more than nine in ten federal leaders say that edge solutions are very or extremely important to meeting mission needs.
- Sixty-five percent of U.S. federal executives report that they have significantly or exponentially increased their deployment of IoT and edge devices over the past three years.
- And eighty-five percent of federal IT decision-makers agree that government can do more to embrace AI technologies on the outer reaches.
Federal agencies need the ability to parse increasing amounts of data from disparate networks of devices – to reliably provide advanced insight no matter where the mission goes. Edge AI can provide these capabilities.
Two key areas of impact
Edge AI will eventually impact most federal operations, but we see two areas as being particularly fit for transformation:
1. Logistics
Supply chains are inherently distributed and decentralized networks with many individual moving processes. Given this, edge AI is a logical tool to support decision-making. In fact, Gartner predicts that 25% of supply chain decisions will be made across intelligent edge ecosystems through 2025. With edge AI, agencies can unlock new supply chain efficiencies and outcomes.
The technology can support widespread data collection and real-time analysis and decision-making. Most data can be processed where the insight is needed most, in near real-time and without the cost of shuttling and storing data in a central cloud. If we look to the far future, edge AI will be key to enabling supply chain operations that are fully transformed by widespread use of intelligent systems such as smart robotics, self-driving trucks, and more.
2. Cybersecurity
As information technology and operational technology ecosystems continue to converge, enterprises are rightfully seeing cybersecurity as much more than a static layer or step in their technology architectures. Rather, cybersecurity is being embedded throughout, to ensure it is responsive and agile in the face of threats that change daily.
Yet, embedded cybersecurity generates data – and a lot of it. With the need to orchestrate and rapidly respond to so much data, edge AI will emerge as a key accelerator. Much like an edge personal assistant such as Alexa, Siri, or Google Assistant provides hyper-personalized service, you can imagine an edge cybersecurity solution in a zero-trust environment providing hyper-local security improvements, while aggregating threat data centrally.
At the same time, though, widespread networks of edge devices expand the available attack surface. Without a strategic approach, this can increase cybersecurity risks. Holistic security frameworks, such as zero trust, as well as rigorous security protocols, routine maintenance, and workforce training, are essential to secure against threats.
Where to begin
To successfully implement edge AI, start with your business architecture and mission goals. Ask yourself – what am I looking to build and why? From there, define what data you need to analyze and where does the mission value for that data sit – near, far, or both? That should drive decisions around enabling central or local access.
Edge AI will require discussion of cost management, operational risk, and business operations. As you work to integrate data and bring it to the edge, you must balance financials and operations, all the while building user trust at every step of the process.
Ultimately, edge AI solves for more than just network and latency issues. With the right approach, it can enable a more strategic framework for how centralized computing and edge devices intersect and deliver mission dominance – providing greater mission ROI and benefits, both near and far.
Authors:
-
Bill Marion | Managing Director, Defense Portfolio Growth & Strategy Lead and Air & Space Force Lead | LinkedIn
-
Todd Massengill | Managing Director, National Security Portfolio | LinkedIn
-
Ian McCulloh, Ph.D. | Managing Director and Chief Data Scientist | LinkedIn
-
Matt Teschke | Associate Director, Machine Learning | LinkedIn
This article is part of a series by Accenture Federal Services on the top four trends building the future of federal AI. Read our overview to see all the trends and stay tuned for our final deep dive in the coming weeks.
This content is made possible by our sponsor, Accenture. The editorial staff of GovExec was not involved in its preparation.
NEXT STORY: Postmodern ERP as the New Normal