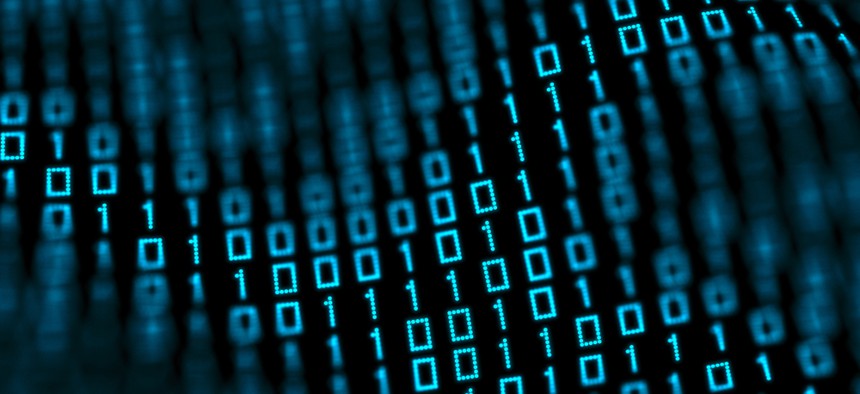
Sur/Shutterstock.com
Got Big Data? First Define Your Big Question
Simple, yet analytical, data thinking can reveal the size of the problem.
Big Data is big. Really BIG. Indeed, the definition from the McKinsey Global Institute, which coined the phrase “big data,” is “data sets whose size is beyond the ability of typical database software tools to capture, manage and analyze.” Big Data is so big that your organization (almost by definition) cannot cope with it.
If, however, your organization does have big software, it might be able to mine some big data for some analytical nuggets. Such data mining, to again quote from McKinsey, is “a set of techniques to extract patterns from large data sets by combining methods from statistics and machine learning with database management.”
But what kind of patterns might your organization seek to extract? If you are looking for crime patterns in your city, you don’t start with sophisticated software. For policing, as CompStat illustrated, an excellent first-order analytical tool is dots on a map. When the data are presented this way, you don’t need a degree in statistics to interpret them.
About a decade ago, I was at a party with a bunch of young quants. They were getting (or had already gotten) their Ph.D.s from MIT or Harvard in some quantitative discipline. One of these Ph.D.s had deserted his intellectual field to work for a supermarket chain. He was charged with mining all of the chain’s data on sales and product placement to determine where in its stores to display which products. For example, which ones should be given those priority spaces at the end of which aisle? To answer this question, the chain had lots of data and lots of computers.
I confess that I thought this analytical task had a very low meaning quotient. I long ago figured out that every grocery store puts the milk at the very back. Everyone needs milk. Indeed, some people come into the store for the single purpose of buying milk. And, if in doing so, they walk past cookies or soup they might make an impulse purchase.
But notice: For this chain’s effort to mine its big data, it had already defined its big question.
But how do we go mining for something that we don’t know is there? For something that we may not know exists? Before people go data mining, they have to do serious data thinking.
During World War II, the allies were analyzing the bullet-hole data from bombers returning from missions over continental Europe. The analysts were not, however, randomly mining the data. They were trying to answer a specific question: How could they improve these planes’ survivability? What parts of the aircraft should they reinforce with armor?
All of the analysts observed where the planes had been hit: primarily on the wings and the tail. So they recommended reinforcing these sections. Like Sherlock Holmes’ Watson, they could see, but they did not observe.
One statistician, however, dissented. Abraham Wald observed that the data came only from the planes that returned. These were not, however, the only planes that took off. Some had failed to return. Why?
Wald was the Sherlock Holmes of this analytical team. He noted that the returning planes did not have many bullet holes in the engines or core fuselage. Assuming that the axis artillery wasn’t very accurate—that their hits on allied airplanes were essentially random—Wald reasoned that the planes that failed to return were the ones that had been hit in the fuselage and engines.
Yes. Wald was “mining” the data. But to do that intelligently, he first had to think. And once he had done his thinking, he didn’t need a big computer to mine big data. For the important data were not the locations of the holes that were captured in some big data set. The key data were where the holes “that didn’t bark.”
As is almost always the case: Data thinking is much more important than data mining. And such thinking always starts with purpose: What are we trying to accomplish? Sell cookies and soup? Save planes and pilots?
Often, data thinking starts with small data. What patterns do we observe in a few data points? What patterns might we observe if we add more data? What did we learn from the few data points? What might we learn if we looked at different data?
What is a big number? A small number? Some short division with a few data points may be revealing. Simple, yet analytical, data thinking can reveal the size of the problem. Or the nature of the problem. Simple, yet analytical, thinking can suggest in what mine to look for what ore.
The supermarket chains are lucky. They know precisely what they want to accomplish. They have been pursuing this objective for a long time. They have accumulated lots of data. And they have people who have been thinking about these data. Thus, they know what questions their mining of their big data might answer.
Before you go mining big data, you have to think analytically with some small data. It’s data thinking that can prove to be really big.
Robert D. Behn, a lecturer at Harvard University’s John F. Kennedy School of Government, chairs the executive education program Driving Government Performance: Leadership Strategies That Produce Results. His book The PerformanceStat Potential will be published by Brookings in 2014. (Copyright 2014 Robert D. Behn)
(Image via Sur/Shutterstock.com)
NEXT STORY: Lawmaker to Feds: You Don’t Need So Many Limos